Identifying Field Boundaries and Classifying Land Cover: An Overview of EOSDA‘s Approach
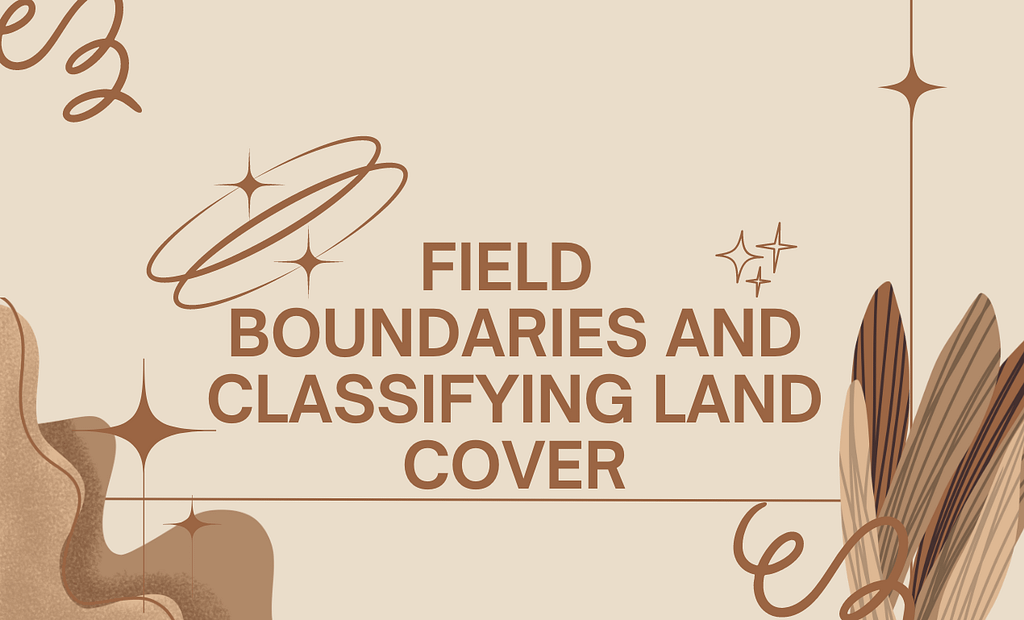
EOSDA Agricultural and environmental monitoring has seen significant advancements with the integration of satellite imagery and advanced machine learning algorithms. One company at the forefront of this technological revolution is EOS Data Analytics (EOSDA). Their approach to identifying field boundaries and classifying land cover is transforming how we understand and manage agricultural landscapes. This blog will delve into EOSDA’s innovative methods, highlighting the techniques and technologies that enable precise field boundary identification and accurate land cover classification.
The Importance of Field Boundary Identification and Land Cover Classification in EOSDA
Field boundary identification and land cover classification are crucial for several reasons:
- Accurate field boundaries help farmers optimize field management practices, such as crop rotation, irrigation, and fertilization, leading to increased yield and reduced environmental impact.
- Environmental Monitoring:
- Understanding land cover types and their distribution is essential for monitoring ecosystem health, biodiversity, and the impacts of climate change.
- Resource Allocation:
- Governments and organizations can better allocate resources and plan land use with precise information about field boundaries and land cover.
- Compliance and Reporting:
- Farmers and land managers can ensure compliance with agricultural policies and regulations and accurately report land use and crop types.
EOSDA’s Approach to Field Boundary Identification
EOSDA utilizes a combination of satellite imagery, machine learning, and geospatial analysis to identify field boundaries with high precision. Here’s an overview of their approach:
- Satellite Imagery:
- EOSDA leverages high-resolution satellite images from sources like Sentinel-2 and Landsat-8. These images provide detailed views of the Earth’s surface, capturing crucial information about vegetation, soil, and water bodies.
- Image Preprocessing:
- Before analysis, satellite images undergo preprocessing steps such as radiometric correction, atmospheric correction, and noise reduction to ensure data accuracy and consistency.
- Segmentation Algorithms:
- Advanced image segmentation algorithms are applied to delineate field boundaries. These algorithms analyze the spectral, spatial, and temporal characteristics of the imagery to detect edges and boundaries of agricultural fields.
- Machine Learning Models:
- Machine learning models, particularly convolutional neural networks (CNNs), are trained on labeled datasets to recognize and classify different types of field boundaries. These models learn to distinguish between various land cover types, such as crops, forests, and urban areas, based on their unique spectral signatures.
- Geospatial Analysis:
- Geospatial analysis tools are used to refine the identified boundaries and integrate them with existing geographic information system (GIS) data. This ensures that the boundaries are accurate and aligned with real-world coordinates.
Classifying Land Cover with EOSDA
Land cover classification involves categorizing the Earth’s surface into different classes, such as forests, grasslands, urban areas, and water bodies. EOSDA’s approach to land cover classification combines remote sensing data with sophisticated classification algorithms. Here’s how they do it:
- Spectral Analysis:
- Different land cover types have distinct spectral signatures. EOSDA analyzes the spectral bands of satellite imagery to identify these signatures. For example, healthy vegetation reflects more near-infrared light, while water bodies absorb most visible and near-infrared light.
- Temporal Analysis:
- Temporal analysis involves studying changes in land cover over time. EOSDA uses time-series data to monitor seasonal variations and long-term changes, which helps in distinguishing between different land cover types that may appear similar at a single point in time.
- Supervised Classification:
- Supervised classification techniques, such as Support Vector Machines (SVM) and Random Forests, are employed to classify land cover. These techniques rely on training datasets with known land cover types to build models that can predict the classes of unknown pixels.
- Unsupervised Classification:
- Unsupervised classification techniques, like K-means clustering, group pixels into clusters based on their spectral properties without prior knowledge of land cover types. These clusters are then interpreted and labeled based on ground truth data and expert knowledge.
- Validation and Accuracy Assessment:
- To ensure the reliability of land cover classifications, EOSDA conducts rigorous validation using ground truth data and independent reference datasets. Accuracy assessment metrics, such as overall accuracy, producer’s accuracy, and user’s accuracy, are calculated to evaluate the performance of classification models.
Applications of EOSDA’s Field Boundary and Land Cover Classification
- Precision Agriculture:
- Farmers can use detailed field boundary maps and land cover classifications to implement precision agriculture techniques, optimizing inputs like water, fertilizer, and pesticides to enhance productivity and sustainability.
- Environmental Conservation:
- Conservationists and environmental agencies can monitor land cover changes to protect natural habitats, manage forests, and track the impacts of human activities on ecosystems.
- Urban Planning:
- Urban planners can utilize land cover data to guide sustainable development, manage green spaces, and plan infrastructure projects in a way that minimizes environmental impact.
- Disaster Management:
- Accurate land cover information helps in assessing the extent of natural disasters, such as floods and wildfires, enabling timely and effective response and recovery efforts.
- Policy and Regulation:
- Governments can use field boundary and land cover data to enforce agricultural policies, support subsidy programs, and ensure compliance with environmental regulations.
Table of Contents
Conclusion
EOSDA’s approach to identifying field boundaries and classifying land cover exemplifies the power of combining satellite imagery with advanced machine learning and geospatial analysis. By providing accurate and up-to-date information about agricultural fields and land cover, EOSDA is enabling better decision-making in agriculture, environmental management, urban planning, and beyond. As technology continues to evolve, the potential for even more precise and comprehensive monitoring of our planet’s surface becomes increasingly attainable, paving the way for smarter and more sustainable management of our natural and built environments.
1 thought on “Identifying Field Top Best Boundaries and Classifying Land Cover: An Overview of EOSDA’s Approach”